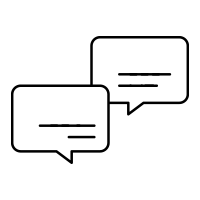
11 Jun How Credible is ChatGPT?
By the i2k Connect AI Expert Team, June 10, 2023
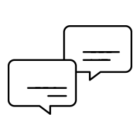
The much-publicized ChatGPT engine is controversial because it is so good at what it does. But what does it do and can we believe what it says?
Large language models generate human-like text using predictive models indicating which letters or words are most likely to follow other ones. Think about your email address book’s auto-fill feature. With only three names starting with John in your address list, your email program will auto-fill one of the three corresponding last names, Jones, Nelson, or Smith, to correspond to typed first name John, probably based on how frequently you’ve emailed each one in the past, or perhaps which one you most recently emailed. The auto-fill doesn’t know who you’re intending to write to, nor does it know the content of the email from which it might deduce the most likely recipient, it’s simply choosing from among three possible recipients with first name John, based on a likelihood it has been programmed to prioritize. Now imagine doing this for the entire English language and your “address book” of choices is the entire text of the internet. In a nutshell, that’s ChatGPT.
Don’t get us wrong, it’s pretty heady stuff. A recent article in the Wall Street Journal nicely summarizes how the program works and its much-publicized major weakness:
“ChatGPT can prioritize among billions of data points to select the single set of 200 words that is most relevant (or will appear most relevant to a human reader). In its own words, it makes probabilistic judgments about future outcomes, blending information from discrete domains into an integrated answer. . . It imitates other writing without copying it. Even though the model is incapable of understanding in the human sense, its outputs reflect an underlying essence of human language.” [“ChatGPT Heralds an Intellectual Revolution,” Kissinger, Schmidt, & Huttenlocher, 2/24/23]
The article also summarizes a well-recognized strength:
“To the naive user, it seems like a supremely fast and highly articulate librarian-scholar coupled with a professorial savant. It facilitates the summary and interrogation of the world’s knowledge far more effectively than any existing technological or human interface, and it does so with unique comprehensiveness.”
To make use of this strength, it will be necessary to provide credibility to the program’s answers. ChatGPT is not transparent about its sources of information or its methods of assimilating those sources, its responses aren’t necessarily reproduceable or explainable, and it’s not above making stuff up in an effort to achieve its prime objective of answering users’ questions. In other words, while its answers are well written, sound logical, and are produced tremendously quickly, their accuracy and veracity will always be in doubt. But because the program is designed to interact with us in a natural-sounding, human-mimicking way, users are inclined to place more credence on the responses than scientific reasoning, or basic common sense, would dictate. ChatGPT is a Greek sophist available world-wide and on-demand, crafting convincing rhetoric with no regard for its veracity.
To demonstrate credibility, the technology has to know more than it does today.
More than 2,000 years ago, Plato identified a specific criterion for deciding that a person knows something or is merely spinning an opinion: he or she can explain (“give an account” of) the fact in question. ChatGPT and other programs like it need to communicate what they do and do not know, and how prior knowledge supports a present answer.
Curiously, ChatGPT can offer explanations of its own reasoning. Consider cases in which ChatGPT gives a ridiculous answer but doubles-down when challenged. (We note that these cases, while they are numerous, are not typical. If they were typical, ChatGPT would be useless, which we do not believe.)
For example, if we ask, “Alice gets off work at 4pm and Bob gets off work at 5pm. Do their schedules overlap?” we first received the response, “Yes, their schedules overlap for one hour from 5pm to 6pm.” And if we regenerate the response, we get, “Alice gets off work at 4pm and Bob gets off work at 5pm, so there is a one-hour gap between their work schedules. Therefore, their schedules do not overlap.”
OpenAI, the creators of ChatGPT, have published a “cookbook” containing suggestions about how to make ChatGPT more “reliable.” One suggestion, which apparently enables GPT-3 (the predecessor to ChatGPT) to improve its ability to solve math problems from 18% accuracy to 79% accuracy, is to ask the chatbot to “think step-by-step.” It produces its reasoning one step at a time. This often works because each step is a smaller logical leap, thus the model is more likely to get it right. Furthermore, it can see each step it generated previously as it generates the next step, so if its logical conclusions on earlier steps are accurate, it’s more likely its conclusions on later steps will be accurate.
For our example, ChatGPT indeed generates a step-by-step explanation if we ask it to “think step-by-step”:
To determine if Alice and Bob’s schedules overlap, we need to compare their work hours and see if there is any time when they are both working. Here are the steps to figure out if their schedules overlap:
-
Determine Alice’s work schedule: We need to know when Alice starts and ends her workday to see if there is any overlap with Bob’s schedule. Let’s say Alice starts work at 9am and ends at 4pm.
-
Determine Bob’s work schedule: Similarly, we need to know when Bob starts and ends his workday. Let’s say Bob starts work at 10am and ends at 5pm.
-
Compare the schedules: We can now compare the two schedules to see if there is any overlap. Alice’s workday ends at 4pm, which is one hour earlier than Bob’s workday. Therefore, there is no overlap between their schedules.
In conclusion, Alice and Bob’s schedules do not overlap since Alice gets off work one hour earlier than Bob.
Steps 1 and 2 make good assumptions about the start times of Alice and Bob. But step 3 is illogical. If we regenerate its response, we get a few variations: one time it concluded we cannot answer definitively if their schedules overlap because we do not know when they start working; another time it assumed Alice worked until 5pm and therefore their schedules do overlap; and another time it stated again that their schedules only overlap one hour, 4-5pm.
Laptop with virtual chat application being used by a business person.
Screen shot of ChatGPT being used on a laptop.
As a toy, this exploration is intriguing and fun. As a tool, perhaps part of an automated workflow that processes emails and, in this case, determines if two employees’ times overlap based on what was written about Alice’s and Bob’s schedules, ChatGPT is so unreliable as to be dangerous in such a role.
Our purpose here is not to demonstrate that ChatGPT can make false statements. This topic has already been addressed by numerous writers in the few months that ChatGPT has been widely available. Our purpose is to determine if ChatGPT can be believed, if it is credible. To be credible, one must be able to explain one’s reasoning. We have seen that ChatGPT is willing to do so, but its reasoning was broken in this case.
We have no other means to judge ChatGPT’s credibility, aside from asking it to explain its reasoning. We cannot examine its internals to see how it came to its conclusions. In fact, ChatGPT does not reason about anything at all—any more than auto-complete of an email address “reasons” about your intended recipient. Another example better illustrates this point.
We asked ChatGPT, “Is a pound of feathers or two pounds of bricks heavier?” ChatGPT responds, “The weight of a pound of feathers and two pounds of bricks is the same, which is one pound.” This is clearly ridiculous, but this output tells us that the vast statistical knowledge ChatGPT possesses about language and its use is somehow still insufficient to prioritize “one pound” and “two pounds” over the mention of “feathers” and “bricks.” If we change “feathers” to “coal” and “bricks” to “diamonds,” ChatGPT responds with a claim that they have different weights but the same mass, and in any event, when in zero-gravity, they would both have the same weight of zero. If we change “coal” to “iron” and “diamonds” to “copper,” we get a proper response (two pounds of copper is heavier than one pound of iron).
Note, if we ask ChatGPT to regenerate its responses to these questions, we essentially receive the same answers, though a small percentage of the time it produces a reasonable response about feathers and bricks (that two pounds are heavier than one). On the other hand, responses about iron and copper are almost always reasonable. In short, there’s something about feathers and bricks, and coal and diamonds, that confuse it, while iron and copper typically do not. We hypothesize that the kinds of text (across the vast internet) that mention comparisons of weight or mass of feathers and bricks or coal and diamonds is more variable, involving more hypotheticals (“if we drop a feather and a brick in zero gravity…”), or includes more descriptions of transitions (“coal turns into diamond…”). We hypothesize that ChatGPT doesn’t know anything about mass, weight, feathers, or bricks. It just knows patterns of language.
But ChatGPT will purport to explain its baseless reasoning. Regarding the “equal weight” of two pounds of feathers and a one-pound brick, in one response it stated,
“Although it may seem counterintuitive, a pound of feathers and two pounds of bricks weigh the same. The weight of an object is determined by its mass, and one pound of feathers has the same mass as two pounds of bricks. This is because a pound is a unit of weight or force, and it is defined as the amount of force required to accelerate a mass of one pound at a rate of 32.174 feet per second squared.”
Is this an explanation? It has some convincing properties. First, it reminds us that the supposed answer is “counterintuitive,” so we should hold our incredulity at the door. Second, it includes facts of science such as how weight is distinct from mass. Furthermore, it cites a law of physics (the gravitational constant). It provides a causal chain, “This is because…” As far as explanations go, it has all the right properties. It’s just wrong.
Although reasoning errors like these are being addressed by the developers, our point is that if you don’t already know the answer, you cannot know which answers and explanations to accept.
We worry that ChatGPT will usher in a wide-scale “Gell-Mann amnesia effect,” a phrase coined by Michael Crichton, in which people will trust ChatGPT’s explanations for any question about a field in which they are not an expert even though they can recognize obvious errors in ChatGPT’s responses for questions in which they are an expert. People might well believe anything ChatGPT produces as long as it has an aura of an expert’s considered explanation—while exhibiting some kind of amnesia about the cases they have likely witnessed where ChatGPT was absurdly wrong. If it is wrong sometimes, and we recognize that, will we know it is wrong other times when we do not have the expertise to recognize it?
Because ChatGPT is only sometimes wrong and is often right, work is under way to tune it with tricks like “prompt engineering,” to force it to improve the accuracy of its answers. With this trick, the developer, OpenAI, suggests ways to massage ChatGPT into being more reliable. It is noteworthy that it is not yet clear if you already must be an expert in the field in which you’re asking for answers to create prompts that produce accurate answers.
A different approach for generating credible inferences with AI programs is possible, indeed well-understood and much used. The approach is grounded in the idea that computer users have more trust in a program’s results when they know what knowledge the AI system is—and is not—using to produce its insights, and they can examine how it is using the knowledge. When that background knowledge can be examined to see that it is nuanced, unbiased, and factual, it becomes a credible ingredient for decision-making and action.
For almost sixty years, AI researchers have built the field of knowledge-based computing on the use of explicit representations of task-specific knowledge (e.g., conditional rules, or statements in formal logic). In other words, a human scientist, or even another AI program, can examine the body of knowledge being used by a knowledge-based platform, evaluate its effectiveness, and reason about its use. That is, the knowledge base can be examined and verified before it is used, and the program’s conclusions are verifiable and analyzable after the fact.
One of the lessons from the first decades of AI is that when AI programs are used for specific, well-bounded tasks, they can be useful “intelligent assistants” backing up their human partners. By providing access to more information and keeping track of more contingencies than their human partners can, they allow people to integrate humanitarian and social concerns into empathetic solutions.
In this partnership model, ChatGPT and other AI programs can be combined with a person’s humanitarian values and judgment—with both backed up by an intelligent assistant accessing subject-matter experts’ accumulated knowledge. When that knowledge is explicitly represented, the assistant can show why GPT’s extracted datapoints or even whole documents satisfy a human query, not just imply that they do. When we give up the idea that AI systems will know everything about everything we gain the possibility of using what they do know to make them credible assistants.
We know we have only begun. However, when an AI program’s performance is dependent on explicitly represented knowledge, users can ask for explanations and reasons and thereby receive “an account” from the system, in Plato’s terms. That is, an AI system can actually know something (many things, not all things). And in well-bounded subject areas, an AI program doesn’t have to pretend to know when it doesn’t because it will be able to show where the gaps in its knowledge lie. Indeed, as Plato points out, knowing what you don’t know is also essential to “knowing” something.
Is ChatGPT useful? What use is an AI system that doesn’t know anything but has lots to say? It is certainly inspiring: we just spent about two thousand words exploring its impact and influence on the human enterprise. We wouldn’t trust it to build a bridge, but it can generate new designs the world has never seen. Some may be useful. ChatGPT is trained on much of the world’s text (some of it factual), and it regurgitates it back in combinations and re-factorings that we might never have thought of ourselves. It is a useful chaos engine—guided by probabilities but not beholden to reason, natural law, or the truth. It’s the court jester who appears “wise enough to play the fool.” It makes us uneasy, it makes us think twice, it makes us more human by showing us the opposite. It’s useful if we’re wise enough to know when not to believe it.
About the Authors
Bruce Buchanan, Ph.D., taught AI at Stanford and the University of Pittsburgh for many years. He is a Fellow and Past President of the Association for the Advancement of Artificial Intelligence and a member of the National Academy of Medicine and the American College of Medical Informatics.
Joshua Eckroth, Ph.D., is an Associate Professor of Computer Science at Stetson University, Associate Editor of AI Magazine, and Editor-in-Chief of AITopics.org.
Eric Schoen, Ph.D., spent many years as a research scientist, software developer, and architect at Schlumberger (now SLB). He is currently Chief Technology Officer at i2k Connect, Inc.
Reid G. Smith, Ph.D., is a Fellow of the Association for the Advancement of Artificial Intelligence and the American Association for the Advancement of Science. He is also an Institute for Electrical and Electronics Engineers Computer Society Distinguished Contributor.
All authors are working at i2k Connect Inc. on applying AI to problems of knowledge management.